How Apple is making QuickType smarter with Machine Learning
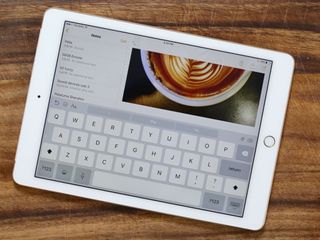
Can Global Semantic Context Improve Neural Language Models? I don't know, but that's the question asked and answered in the latest entry on Apple's Machine Learning Journal.
From Apple:
Today, most techniques for training word embeddings capture the local context of a given word in a sentence as a window containing a relatively small number of words (say, 5) before and after the word in question—"the company it keeps" nearby. For example, the word "self-evident" in the U.S. Declaration of Independence has a local context given by "hold these truths to be" on the left and "that all men are created" on the right.In this article, we describe an extension of this approach to one that captures instead the entire semantic fabric of the document—for example, the entire Declaration of Independence. Can this global semantic context result in better language models? Let's first take a look at the current use of word embeddings.
It's heady stuff but a good read for anyone interested in how Apple is working to make Siri and systems like QuickType better.
Master your iPhone in minutes
iMore offers spot-on advice and guidance from our team of experts, with decades of Apple device experience to lean on. Learn more with iMore!
Rene Ritchie is one of the most respected Apple analysts in the business, reaching a combined audience of over 40 million readers a month. His YouTube channel, Vector, has over 90 thousand subscribers and 14 million views and his podcasts, including Debug, have been downloaded over 20 million times. He also regularly co-hosts MacBreak Weekly for the TWiT network and co-hosted CES Live! and Talk Mobile. Based in Montreal, Rene is a former director of product marketing, web developer, and graphic designer. He's authored several books and appeared on numerous television and radio segments to discuss Apple and the technology industry. When not working, he likes to cook, grapple, and spend time with his friends and family.