How 'Hey Siri' Works
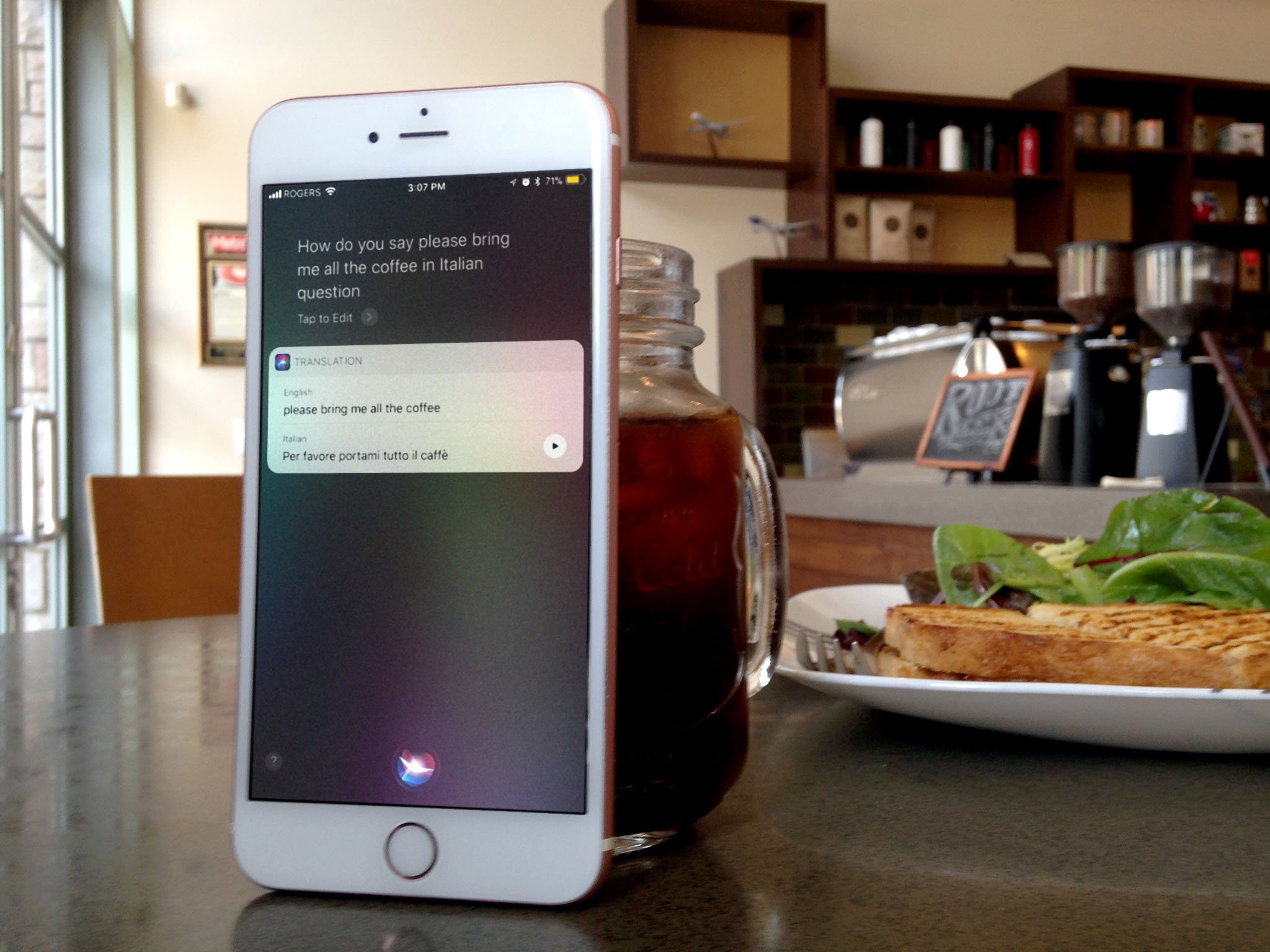
Last fall, Apple's Machine Learning Journal began a deep dive into 'Hey, Siri', the voice trigger for the company's personal digital assistant. (See below.) This spring, the Journal is back with another dive into how it tackles not only knowing what is said but who said it, and how it balances imposter acceptance vs. false rejections.
From Apple:
The phrase "Hey Siri" was originally chosen to be as natural as possible; in fact, it was so natural that even before this feature was introduced, users would invoke Siri using the home button and inadvertently prepend their requests with the words, "Hey Siri." Its brevity and ease of articulation, however, bring to bear additional challenges. In particular, our early offline experiments showed, for a reasonable rate of correctly accepted invocations, an unacceptable number of unintended activations. Unintended activations occur in three scenarios - 1) when the primary user says a similar phrase, 2) when other users say "Hey Siri," and 3) when other users say a similar phrase. The last one is the most annoying false activation of all. In an effort to reduce such False Accepts (FA), our work aims to personalize each device such that it (for the most part) only wakes up when the primary user says "Hey Siri." To do so, we leverage techniques from the field of speaker recognition.
It also covers explicit vs. implicit training: Namely, the process at setup and the ongoing process during daily use.
The main design discussion for personalized "Hey Siri" (PHS) revolves around two methods for user enrollment: explicit and implicit. During explicit enrollment, a user is asked to say the target trigger phrase a few times, and the on-device speaker recognition system trains a PHS speaker profile from these utterances. This ensures that every user has a faithfully-trained PHS profile before he or she begins using the "Hey Siri" feature; thus immediately reducing IA rates. However, the recordings typically obtained during the explicit enrollment often contain very little environmental variability. This initial profile is usually created using clean speech, but real-world situations are almost never so ideal.This brings to bear the notion of implicit enrollment, in which a speaker profile is created over a period of time using the utterances spoken by the primary user. Because these recordings are made in real-world situations, they have the potential to improve the robustness of our speaker profile. The danger, however, lies in the handling of imposter accepts and false alarms; if enough of these get included early on, the resulting profile will be corrupted and not faithfully represent the primary users' voice. The device might begin to falsely reject the primary user's voice or falsely accept other imposters' voices (or both!) and the feature will become useless.
In the previous Apple Machine Learning Journal entry, the team covered how the 'Hey Siri' process itself worked.
A very small speech recognizer runs all the time and listens for just those two words. When it detects "Hey Siri", the rest of Siri parses the following speech as a command or query. The "Hey Siri" detector uses a Deep Neural Network (DNN) to convert the acoustic pattern of your voice at each instant into a probability distribution over speech sounds. It then uses a temporal integration process to compute a confidence score that the phrase you uttered was "Hey Siri". If the score is high enough, Siri wakes up.
As is typical for Apple, it's a process that involves both hardware and software.
The microphone in an iPhone or Apple Watch turns your voice into a stream of instantaneous waveform samples, at a rate of 16000 per second. A spectrum analysis stage converts the waveform sample stream to a sequence of frames, each describing the sound spectrum of approximately 0.01 sec. About twenty of these frames at a time (0.2 sec of audio) are fed to the acoustic model, a Deep Neural Network (DNN) which converts each of these acoustic patterns into a probability distribution over a set of speech sound classes: those used in the "Hey Siri" phrase, plus silence and other speech, for a total of about 20 sound classes.
And yeah, that's right down to the silicon, thanks to an always-on-processor inside the motion co-processor, which is now inside the A-Series system-on-a-chip.
iMore offers spot-on advice and guidance from our team of experts, with decades of Apple device experience to lean on. Learn more with iMore!
To avoid running the main processor all day just to listen for the trigger phrase, the iPhone's Always On Processor (AOP) (a small, low-power auxiliary processor, that is, the embedded Motion Coprocessor) has access to the microphone signal (on 6S and later). We use a small proportion of the AOP's limited processing power to run a detector with a small version of the acoustic model (DNN). When the score exceeds a threshold the motion coprocessor wakes up the main processor, which analyzes the signal using a larger DNN. In the first versions with AOP support, the first detector used a DNN with 5 layers of 32 hidden units and the second detector had 5 layers of 192 hidden units.
The series is fascinating and I very much hope the team continues to detail it. We're entering an age of ambient computing where we have multiple voice-activated AI assistants not just in our pockets but on our wrists, on our laps and desks, in our living rooms and in our homes.
Voice recognition, voice differentiation, multi-personal assistants, multi-device mesh assistants, and all sorts of new paradigms are growing up and around us to support the technology. All while trying to make sure it stays accessible... and human.
We live in utterly amazing times.
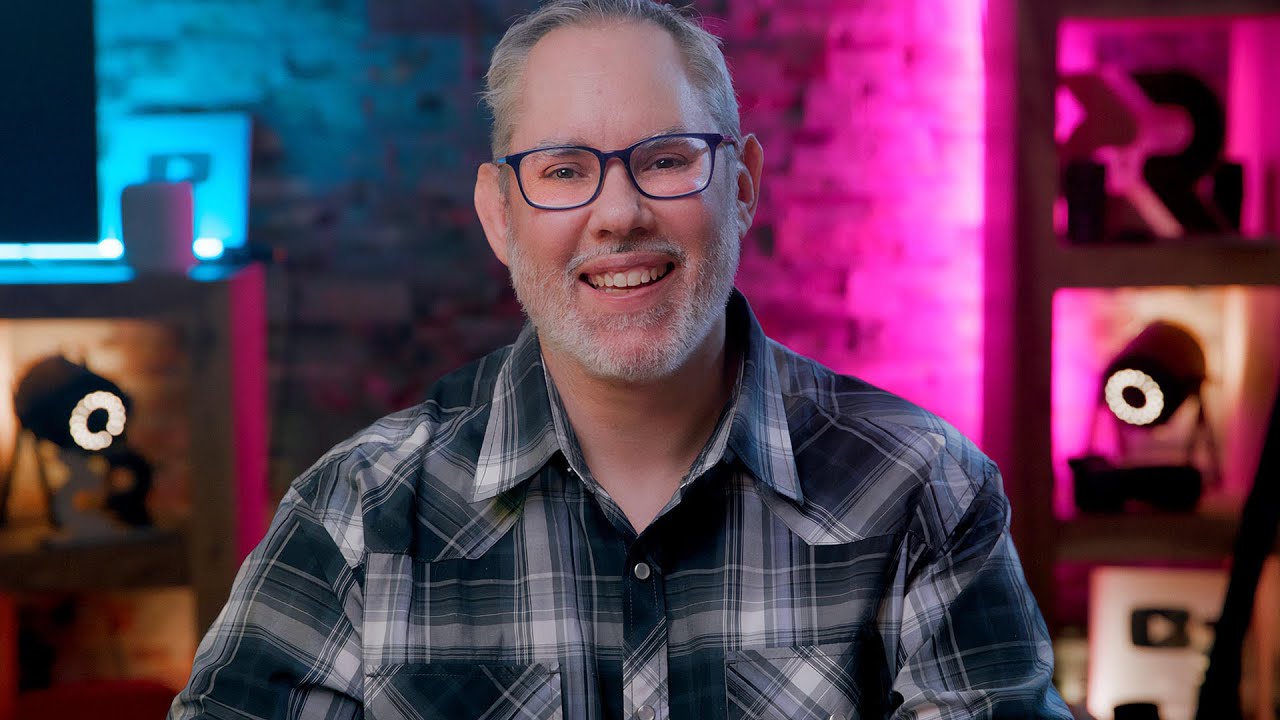
Rene Ritchie is one of the most respected Apple analysts in the business, reaching a combined audience of over 40 million readers a month. His YouTube channel, Vector, has over 90 thousand subscribers and 14 million views and his podcasts, including Debug, have been downloaded over 20 million times. He also regularly co-hosts MacBreak Weekly for the TWiT network and co-hosted CES Live! and Talk Mobile. Based in Montreal, Rene is a former director of product marketing, web developer, and graphic designer. He's authored several books and appeared on numerous television and radio segments to discuss Apple and the technology industry. When not working, he likes to cook, grapple, and spend time with his friends and family.