Taking Siri far-field with HomePod
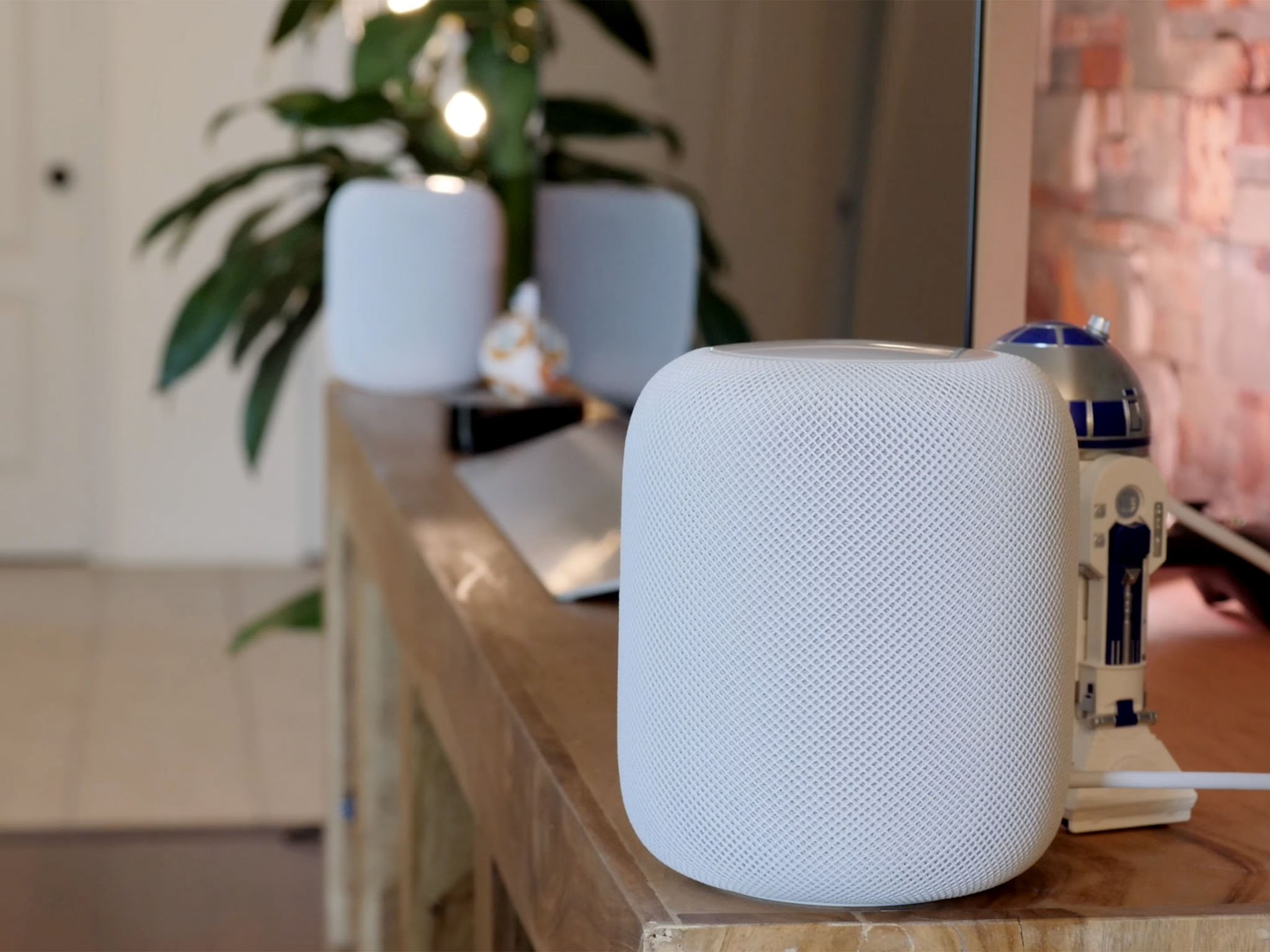
Siri, Apple's personal virtual assistant, debuted on the iPhone but, step by step, expanded to iPad, Mac, and Apple Watch. When it came time to build the HomePod, though, Apple needed to make a version of Siri optimized not for wrist, hand, lap or table distances, but for across the room and around the house. That meant making Siri optimized for far-field use cases.
Apple's Machine Learning Journal:
Far-field speech recognition becomes more challenging when another active talker, like a person or a TV, is present in the same room with the target talker. In this scenario, voice trigger detection, speech decoding, and endpointing can be substantially degraded if the voice command isn't separated from the interfering speech components. Traditionally, researchers tackle speech source separation using either unsupervised methods, like independent component analysis and clustering [4], or deep learning [5, 6]. These techniques can improve automatic speech recognition in conferencing applications or on batches of synthetic speech mixtures where each speech signal is extracted and transcribed [6, 7]. Unfortunately, the usability of these batch techniques in far-field voice command-driven interfaces is very limited. Furthermore, the effect of source separation on voice trigger detection, such as that used with "Hey Siri", has never been investigated previously. Finally, it's crucial to separate far-field mixtures of competing signals online to avoid latencies and to select and decode only the target stream containing the voice command.
Voice assistants are still new by user interface standards but will inarguably play a huge part in the future of human-machine interactions, so I can't get enough of this kind of stuff.
Well worth a read.
iMore offers spot-on advice and guidance from our team of experts, with decades of Apple device experience to lean on. Learn more with iMore!
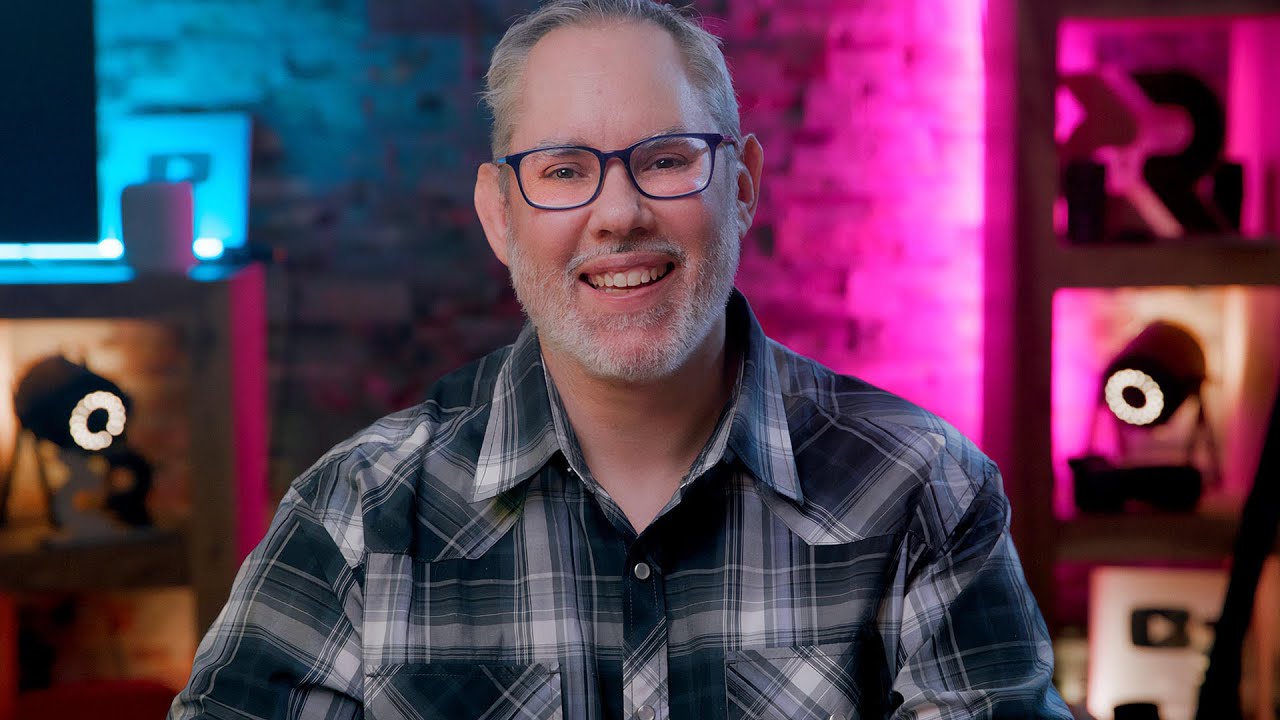
Rene Ritchie is one of the most respected Apple analysts in the business, reaching a combined audience of over 40 million readers a month. His YouTube channel, Vector, has over 90 thousand subscribers and 14 million views and his podcasts, including Debug, have been downloaded over 20 million times. He also regularly co-hosts MacBreak Weekly for the TWiT network and co-hosted CES Live! and Talk Mobile. Based in Montreal, Rene is a former director of product marketing, web developer, and graphic designer. He's authored several books and appeared on numerous television and radio segments to discuss Apple and the technology industry. When not working, he likes to cook, grapple, and spend time with his friends and family.